In recent years, the rapid development of Generative AI (GenAI) has revolutionized various industries from art and music to writing and game design. This innovative technology is reshaping how we create and interact with content. Cybersecurity is no exception. While GenAI presents new opportunities for enhancing security operations, it also comes with significant risks and challenges. As organizations adopt these advanced tools, understanding their potential impacts on cybersecurity is crucial. Let’s explore the pros, cons, and challenges that come with integrating GenAI into cybersecurity.
1. The Pros of Generative AI in Cybersecurity
1.1 Enhanced Threat Detection
One of the most promising applications of Generative AI in cybersecurity is its ability to analyze patterns and detect anomalies. The mute question is how effective is it in identifying new and sophisticated threats? Can AI-generated models stay ahead of evolving cyberattack strategies, or do they risk becoming obsolete as threats become more complex?
Cyber threats evolve rapidly, with attackers constantly devising new tactics to breach systems. GenAI, with its ability to quickly analyze massive amounts of data, can detect anomalies and identify suspicious behavior faster than traditional methods. By recognizing patterns that might go unnoticed by human analysts, GenAI can flag potential threats in real-time. This proactive detection can prevent escalation of security incidents, giving security teams a crucial edge.
1.2. Automated Incident Response
One of the most valuable aspects of GenAI is its ability to automate the response to security incidents. Instead of relying solely on human intervention, AI systems can recommend actions or even execute pre-defined responses to mitigate threats. This dramatically reduces response time, helping organizations contain threats before they cause serious damage. Further, this helps immediately isolate compromised machines—all without waiting for human intervention
1.3 Phishing and Fraud Detection
GenAI models can analyze known malware variants and predict potential future mutations. Phishing remains one of the most common ways attackers compromise systems. GenAI can analyze emails and messages, detecting subtle signs of phishing that might escape human detection. From language patterns to suspicious links, GenAI models can help organizations block fraudulent attempts before they reach employees, reducing the risk of social engineering attacks.
1.4 Improved Security Policy and Configuration Suggestions
GenAI can analyze historical attack data and security incidents to recommend optimized security configurations. This can be particularly useful for organizations that struggle with maintaining effective security policies. By using AI-generated insights, companies can ensure their defenses are up to date and aligned with best practices.
2. The Cons of Generative AI in Cybersecurity
2.1. Weaponization by Cybercriminals
Unfortunately, the same technology that helps secure systems can also be used by cybercriminals. Adversaries can leverage GenAI to generate sophisticated malware, phishing attacks, or even deepfake content. With the help of AI, attackers can craft more convincing social engineering attempts, making it harder for traditional defenses to detect these threats. The rise of AI-generated attacks could create a new class of highly personalized, scalable cyberattacks.
2.2. False Positives and Negatives
While GenAI models can identify threats faster than humans, they can also produce false positives (flagging harmless activity as malicious) or false negatives (failing to detect actual threats). In cybersecurity, false positives can overwhelm analysts with unnecessary alerts, leading to alert fatigue, while false negatives can allow dangerous attacks to slip through undetected. The balance between precision and recall remains a challenge for AI-driven systems.
2.3. Vulnerabilities in AI Systems
Just as GenAI is used to protect systems, it can also be vulnerable to adversarial attacks. Cybercriminals can trick AI models by feeding them slightly altered inputs (known as adversarial examples), which may cause the AI to misclassify threats. This can result in an attacker bypassing AI-based defenses. Moreover, AI systems can have their own vulnerabilities, creating new attack surfaces that need to be secured.
2.4. Privacy Concerns
Many GenAI systems require large datasets for training, and these datasets may include sensitive or personally identifiable information (PII). In some cases, there’s a risk that AI models could inadvertently expose or misuse this data, leading to privacy violations. Ensuring that AI systems comply with privacy regulations like GDPR and CCPA is a challenge that organizations must address when implementing AI-based cybersecurity solutions.
2.5. Over-Reliance on Automation
While automation is a key strength of GenAI, over-relying on it can be risky. If organizations become too dependent on AI for detecting and responding to threats, they might neglect manual oversight. This could lead to situations where a critical attack is missed, or where the AI’s decisions are not questioned. Human expertise is still crucial for verifying AI-generated insights and making nuanced decisions.
3. The Challenges of Using Generative AI in Cybersecurity
3.1. Data Availability and Quality
GenAI models rely heavily on high-quality, labeled data for training. In cybersecurity, obtaining such data is a challenge, especially for rare or emerging threats. Additionally, training data must be comprehensive enough to cover various attack vectors. If the model isn’t trained on diverse and high-quality data, its effectiveness is reduced.
3.2. Explainability and Transparency
One of the key challenges with AI, especially deep learning models, is the “black box” nature of their decision-making processes. Security professionals need to trust the AI’s output, but without clear explanations, this trust can be hard to establish. If a GenAI system flags an activity as malicious, but can’t explain why, security teams may struggle to act on that information. Explainable AI (XAI) is an emerging field that seeks to address this issue, but it remains a significant challenge in cybersecurity.
3.3. Adapting to New Threats (Model Drift)
Cyber threats evolve rapidly, and AI models that were effective at one point may become less effective over time. This phenomenon, known as model drift, occurs when the AI’s ability to detect threats diminishes as attackers develop new tactics. To maintain effectiveness, GenAI models must be regularly retrained and updated with fresh data, which can be resource-intensive.
3.4. Integration with Existing Security Infrastructure
Implementing GenAI into an organization’s existing cybersecurity infrastructure is not always straightforward. Compatibility issues, integration challenges, and the need for specialized expertise can slow down the adoption of GenAI tools. In some cases, organizations might need to overhaul parts of their security architecture to accommodate AI-driven systems.
3.5. Cost and Resource Requirements
Training and deploying GenAI models, especially large-scale ones, can be expensive and resource-intensive. Not every organization has the financial means to invest in the hardware, software, and talent needed to effectively implement GenAI in their cybersecurity operations. Cloud-based AI services can reduce costs, but for some, the expense remains a significant barrier.
Conclusion
Generative AI is significantly influencing cybersecurity by providing advanced tools for detecting threats, automating responses, and improving vulnerability analysis. Its ability to identify patterns in vast datasets enhances defensive capabilities. However, it also presents risks, such as enabling more sophisticated cyberattacks and the creation of malicious code. The challenges lie in balancing AI’s benefits with the potential for misuse, ensuring robust security measures, and maintaining ethical standards. In summary, while generative AI offers promising solutions for cybersecurity, it requires careful management to mitigate associated risks and challenges.
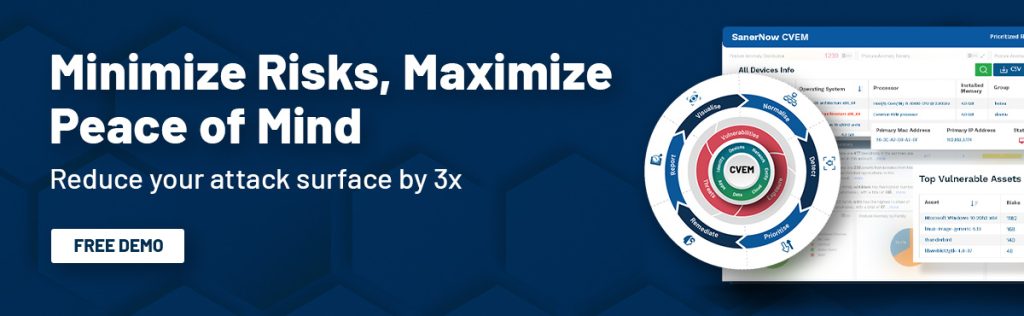