NVIDIA AI Summit is a conference that we eagerly looked forward to. We expected a lot of important NVIDIA announcements to be made. A lot of stakeholders from Governments, Corporations, Academia will come together to discuss current adoption of AI and how it has empowered them. We booked our reservations well in advance and booked air tickets. However, just 2 weeks into the conference, when we were perusing sessions, we got exciting news that keynote will include fireside chat between giants Jenson Huang and Mukesh Ambani. We had to reschedule our flights to attend this seminar, which no one wants to miss. Prior to conference, it has been raining in Bangalore incessantly for 2 weeks and we were anxious that rain will not play spoilsport for our enthusiasm
On October 24, early morning, we started our journey to Bangalore International Airport and luckily there was no rain. We landed in Bombay airport by 9.30 AM and expecting traffic delay we booked a cab to World Jio Center and enroute drop our luggage at the Hotel. We were in utter shock looking at the traffic jam at Bombay and wondering whether the keynote session might have started. We reached the conference around11.15 AM and were relieved to know it had not started. However, we have to stand in the serpentine queue to get our registration badges and then go to the presentation room. By the time we reached there, it was full, and we had to go to another room with a TV monitor.
Jensen’s Keynote Address
Soon Jensen made his appearance and took the entire audience by storm by his personality and vitality. He started off by mentioning that IBM System 360, in 1964, started the IT industry as we know it. They introduced the idea of the general-purpose computing, Central processing unit (CPU), Operating system (OS), multitasking, separation of hardware and application software via OS layer and family compatibility of application software. The customer could benefit from the installation base of hardware to run software applications over a long period of time. This architectural benefit across generations ensured that the investment that you make in software the Investments you make in using the software is not squandered every single time you buy new hardware. In the last 30 years, the hardware speed doubled every year as per Moore’s law in an architecturally compatible manner and software ran at a higher speed without disruption.
Two events, the invention of the system 360 aided by Moore’s law and Windows PC, drove what is unquestionably one of the most important industries in the world. Around the 1990s, the scaling of CPUs has reached its upper limits, and we can’t continue to ride that curve. We no longer can afford to do nothing with software and expect that our computing experience will continue to improve so that costs will decrease. In 1993 NVIDIA started with a vision to accelerate by augmenting general purpose computing by the process of offloading computationally intensive tasks using Cuda programming model. Surprisingly, this acceleration benefit has the same qualities as Moore’s law for software application. Computer Graphics is made possible because of Nvidia introducing the new GPU processor, the first accelerated computing architecture running Cuda. Ever since, over the last 30 years, NVIDIA has been on a journey to accelerate one domain of application after another. The journey was not simple, there was a need to reinvent the computing stack from the algorithms to the architecture underneath to applications at the top level. As of today, CUDA enabled applications cover varieties of domains such as semiconductor manufacturing, computational lithography, simulation, computer-aided engineering, 5G radios, quantum computing, gene sequencing, CuVS (Vector Store) for integrating AI knowledge bases, CuDF for processing data frames, CuOPT to solve optimization problem and CuDNN for AI. Now CUDA acceleration has reached a tipping point.
About a decade ago, NVIDIA, inspired by the effectiveness and success of Alexnet started thinking of using deep learning to reinvent computing. Currently, the method software (called software 1.0) is done is that programmers would code algorithms, which are called functions, that run on a computer to which input is applied to predict the output. This has led to coding and programming becoming one of the largest industries in the world. However, going forward this approach is disrupted. It is now not coding but machine learning algorithms that are used to study the patterns and relationships of massive amounts of observed data to essentially learn from it the nature of function that predicts it. So, we are essentially designing a universal function approximator using machines to learn the expected output that would produce such a function.
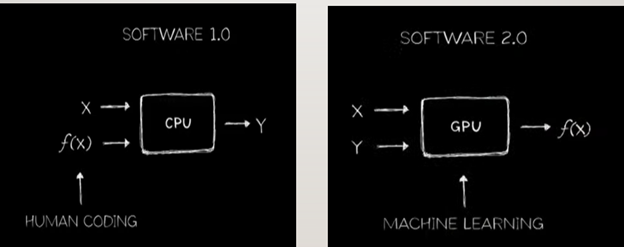
NVIDIA has come up with a kind of AI supercomputer Blackwell, an incredible system that is designed to study data at an enormous scale so that we can discover patterns and relationships and learn the meaning of the data. We have now learnt the representation or the meaning of words and numbers and images and pixels and videos to represent information in so many different modalities and to translate from one modality to another modality. This has led to an explosion in the last 2-3 years in the number of generative AI companies with tens of thousands to tens of billions of dollars investment. This a fabulous, advanced hardware NVIDIA has built that delivers powers with 72 GPUs at one go. This comes with equally fabulous Cuda software CNN software, Megatron for training the LLM, tensor RT for doing uh distributed multi-gpu inference and then on top of that we have two software stacks NVIDIA AI and then the other is Omniverse. Unlike Moore’s law both data and data model are increasing by a factor of 2 every year and this increase is incredible. Further, we continue to find that AI continues to get smarter as we scale up. LLM like ChatGPT demonstrated the power of one-shot learning. This may be simple but going forward intelligence requires thinking and thinking is reasoning one is doing while path planning or simulating in the mind or reflecting on one’s own answers. So thinking results in higher quality answers.
The scaling law of LLM based on empirical studies show that both training data size and model size double with each passing year. This necessitates that the computation has to increase by a factor of 4 to achieve the same efficiency. This needs incredible computing power and AI gets smarter with increased size. NVIDIA has found out a second scaling law at the time of inference that more thinking leads to better answers.
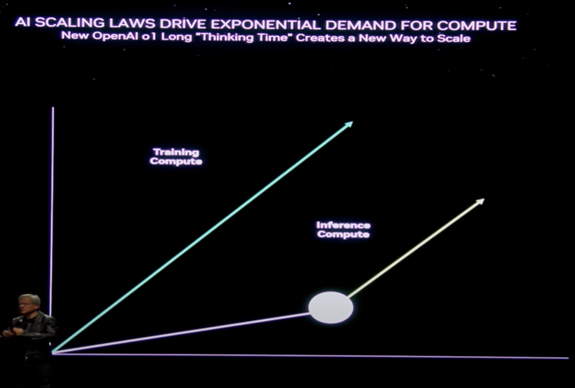
The size of the LLM models is ever increasing and in addition to support multimodality capability, reinforcement learning capability, synthetic data generation capability the amount of data that we use to train these models has really grown tremendously and Blackwell with its super-fast token generation capability can effectively and efficiently handle this compute.
After explaining NVIDIA achievements at length Jensen talked about NVIDIA initiatives in India. He won the heart of the audience by saying that NVIDIA sounds much like “India”, and he liked the aura of mysticism associated with India. He continued, in order to build an AI ecosystem in any industry or in any country you have to have ecosystem of the infrastructure and towards this end NVIDIA together with Yoda, ET Communications and our other partners are joining to build fundamental Computing infrastructure in India. The plan is to increase computing by 20 times by the end of 2024. Further, NVIDIA is working with partners in India to build the Hindi large language model and Hindi with its dialect variants. Finally, NVIDIA has teamed up with its service partners Wipro to Infosys to TCS to take the AI models and the AI infrastructure out to the world’s Enterprises.
Jensen shifted gears to explain agentic AI architecture where different AI agents handle important sub-tasks in coordination with the central reasoning LLM. NVIDIA has come up with a framework, the suite of libraries, called “Nemo” to help create, monitor and run agent lifecycles. These libraries enable agents to be created, onboarded, improved and deployed as per the life cycle of the agent. This work also led to the creation of API inference micro service called “Nim”. He then introduced AI software stacks “NVIDIA AI” and the “Omniverse” and their purpose. He has invited Mishel to tell how they are using NVIDIA offering to build locally and grow globally.
Jensen called to everyone’s surprise Akshay Kumar, a well-known actor from the Bollywood. They had been friends for 30 years and their friendship started in Bangkok. Both are martial art enthusiasts and agreed how It has taught them to be disciplined. Jensen let out a secret of Akshay Kumar that his next movie as a super cop is about to be released. Akshay, not so happy at his secret being revealed, asked Jensen what AI can do that humans cannot do. Jensen in his usual manner said there are lot of things AI cannot do as humans and of the things it can do, it will surpass human. Akshay queried whether AI could take away jobs. Jensen intelligently answered that it cannot take of jobs who can use AI for useful purposes. Jensen also said AI can fall into bad actors for misuse. There are lot of good things happening that will check its misuse.
Then came the conversation between Jensen and Ambani, everyone looked forward to. They started conversation in a lighter note with Mukesh telling that the conference building is built by his wife. Jensen replied that her house is bigger than Mukesh’s house and cracked a joke that from Mukesh’s house he could see his house in California. Then, Mukesh gave his version of what NVIDIA means. He connected NVIDIA with Vidya, which stands for knowledge. He explained, Vidya’s goddess is Saraswati and if we sincerely follow it, Lakshmi, goddess of wealth will automatically come. Jensen quipped that he knew about this 30 years ago.
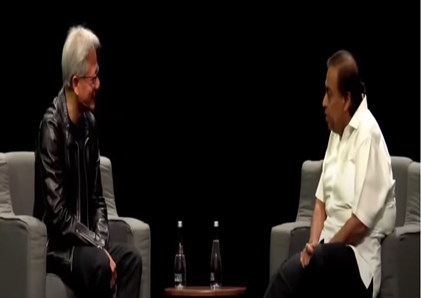
After pleasantries, they discussed about transformative role of AI in India’s development and the potential of India to become a global AI powerhouse. Jensen praised India’s IT sector and highlighted the success of upskilling 200,000 professionals in AI. He asked how they could continue accelerating India’s digital transformation. Mukesh emphasized India’s youthful demographic, which he called a driving force behind the country’s growing digital economy and praised Prime Minister Modi’s vision for a digital society. He described how India has become an innovation hub across sectors, including energy, pharmaceuticals, and tech.
Mukesh further explained that Reliance’s Jio network has revolutionized India’s digital infrastructure, providing affordable data at globally unmatched rates, thus enabling widespread digital inclusion and creating substantial value for Indian consumers. This advancement, he said, lays the foundation for India to lead in AI.
The two leaders announced a partnership between Reliance and Nvidia to build AI infrastructure in India, combining India’s data resources and digital infrastructure with Nvidia’s AI technology. Jensen noted Modi’s foresight in pushing for a national AI infrastructure, so that India could retain control over its data and become self-sufficient in AI, rather than outsourcing intelligence.
Mukesh commended the contributions of open-source AI models, such as Meta’s Llama, which democratize access to advanced AI tools. They expressed hope that these models would empower Indian developers to create a unique AI ecosystem that reflects local needs. Mukesh shared plans to scale up Reliance’s AI infrastructure to deliver AI to all Indians affordably, with a focus on accessibility, even on existing devices.
Jensen and Mukesh closed with a commitment to make India a leader in the AI industry, expressing confidence in India’s potential to surprise the world with innovations in intelligence. They ended with well-wishes for Diwali, celebrating India’s prosperous future in AI/LLM technology.
Niki Parmar’s “Lessons On Transformations”
The next session we attended is “On Transformation” from Niki Parmar, one of the co-authors of the celebrated “Attention is all you need” paper in 2017.
Niki is very excited about her work at Google Brain working on data and modeling and being able to extract information and learn from it. While working with peers on transformers she had a feeling of venturing into the unknown. Niki was thinking about general principles and universal operations that could aid us in developing universal function approximators. Of course, without NVIDIA’s hardware-software ecosystem pushing the boundaries would have been impossible. This also made other bigger things possible.
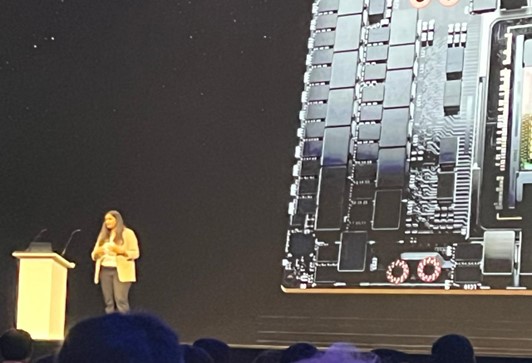
Transformers are one of the inflexion points in 2017. With transformers, they had to think about good representations across many modalities and tasks. They found transformers are versatile and can scale very well and could be applied to diverse modalities like images, proteins, speech, and music, outperforming their counterparts out-of-the box. The core part of transformer is self-attention which helps to learn representations as a function of the context. to learn content-gated representations. Key strengths of transformers include the ability to model hierarchical structure, long-range dependency capture, and parallelizability, which make them both powerful and efficient. Transformer helps in the universal way of approximating a similarity function and therefore universally applicable across many applications. Transformers work very well for different NLP tasks without need for special tuning.
Transformers play a central role, showing improved predictability with increased computation, model sophistication, and data volume. Learning from human demonstrations and preferences helped bridge the gap between humans and AI. The next breakthrough in transformers is refinement of their capabilities through instruction tuning and Reinforcement Learning from Human Feedback (RLHF). Alignment and supervision are ongoing research topics to explore ways to enhance model reasoning, including user feedback, tool integration, self-reflection, and guided principles. The next major step is guided search technique using reward models in multi-step, long reasoning chains to solve complex tasks.
Future directions involve new methods of data collection and curating user interactions, enabling models to learn across varied environments like web browsers, IDEs, and custom applications. A related idea is how to augment search and reasoning capabilities with experts in the loop for solving multi-stage tasks. Experts can come in different point of the workflow and give corrective feebback for right orientation. In addition, there is an emphasis on models that can autonomously explore to acquire data with minimal user supervision.
India’s R&D landscape could be transformed by promoting technology-driven initiatives, massive-scale data and feedback collection, and creating intelligent, scalable solutions in fields like healthcare, education, and efficient model deployment on smaller devices.
Tips for Startups
There was panel discussion regarding “Generative AI—The Next Two Years: Pathways to Innovation and Success from Startups’ which featured founders and investors. Roopan speaking from an investor’s perspective spoke about advantages and opportunities. India has strong AI talent pool. Academia is backing with good research initiatives and India has good semiconductor talent also. Government initiative to support these activities. We need to build hardware based on needs of India not banking on importing from outside. There is an evolving market for AI solutions. The other development of hardware market in India for India. Hemanth, another investor drew attention to the fragmented nature of AI supply chain and cautioned that we are not still at zero marginal cost of intelligence. The areas of focus for benefits and opportunities are data and computing to be harnessed.
As for imperatives Nishit recommendation for startups is to find application for infrastructure and build domain knowledge into apps. Jithendra spoke about startups to think about how to create accurate data. They need to think of improving or building available evaluation strategies relevant to application context. Jithendra felt it is hard for startups to position themselves in the current AI market. At the one end infra-investment is capital intensive and the AI applications which may not be investible business. The space where value can be created is challenging and is too crowded.
India’s Vision for Exascale Computing
This was a panel discussion for India need and vision for exascale computing. It has representatives from UIDAI (Adhaar card), Weather and Health fields. Currently weather monitoring and information generation is a computation intensive process and at present they are not able to generate actionable forecasts. It requires beefing up of computational infrastructure to analyze large amounts of weather data interpret it and generate useful forecasts, which require moving to exascale computing. The expert from UIDAI also explained the need for exascale computing. From the health perspective, a lot of computing power is needed for genome sequencing and managing health data with its privacy and security concerns. There is a need for the development of AI agents across applications to collect data that can be processed with exascale computing. The convergence of exascale computing and HPC convergence would be good for India.
AI Governance with LLM
To support economic, administrative, judicial, and legislative functions, a sovereign Large Language Model (LLM) can be developed with a focus on local languages, cultural values, and compliance with regional laws. Such a model would enable cost-effective inference while keeping cash flow within the local economy and ensuring accessible AI for essential business and governmental operations.
In the administrative domain, virtual assistants could enhance ministry functions by creating multi-agent systems to support specific missions. Citizen service agents could be designed to facilitate information dissemination and compliance with public services.
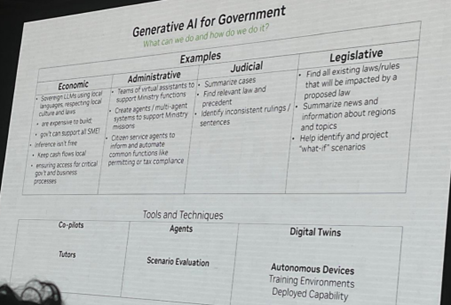
For the judicial sector, an LLM could help summarize cases, identify relevant laws and precedents, and detect inconsistencies in rulings and sentences. In the legislative space, it could identify impacted laws from proposed legislation, provide news summaries on key regions and topics, and assist in exploring hypothetical scenarios for informed decision-making.
LLM Security and Safety
Mohit Sewak started by saying Responsible/Constitution AI is treated as Trustworthy AI in NVIDIA. Although LLM are trained on vast amounts of data to do human-like inference, they are susceptible to hallucinations. They generate wrong answers with an aura of authority. Besides, depending on content of training data they generate biased or toxic or inappropriate content. Further prompt injection attacks are unavoidable and LLMs can be jailbroken or tricked into doing unintended actions. When integrated with unsafe third-party applications they can lead to system compromise. Hence the motivation for using guardrails from safety and security perspective.
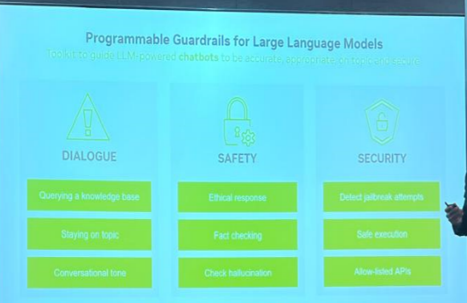
He said LlamaGurad is fine-tuned and has done a major groundwork in identifying important taxonomy classes for classifying inappropriate content and handling them via guardrails. NVIDAI have improved upon Llamaguard and come up with their own AEGIS system for guard rail protection. This provides advanced security measures, including programmable guardrails for LLM-powered chatbots, content safety moderation, and alignment algorithms. More details can be found in 2404.05993v2.
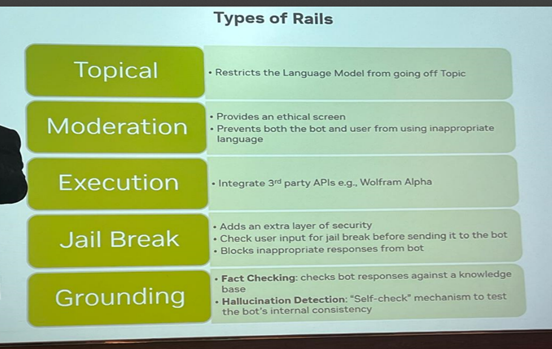
Mohit took the use case of railway booking chatbot and demonstrated techniques of how to use input and output guard rails to enhance safety and security of LLms.. These tools are made Open source for wide adaptation.
NVIDIA AI Summit Expo Section
The conference featured over 30 stalls, and we focused our time on those relevant to GenAI and cybersecurity. At the Shakti Clouds booth, we learned about their tailored offerings for the Indian market and collected contact information for further engagement. The majority of stalls showcased AI and LLM applications, with the NVIDIA booth standing out in particular. Their display featured cutting-edge advancements like Physical AI and Blackwell. An interesting discovery was their use of AI agents, powered by Retrieval-Augmented Generation (RAG) with NIM and Morpheus, to accelerate the triaging of software vulnerabilities. This setup harnesses the power of Generative AI to significantly improve CVE detection and mitigation.